Breaking the Black Box: Understanding why discounts may sometimes lead to higher churn
Menhir Masterclass - Edition #1
"If you offer this customer a discount he will churn."
"Yes, but... why?"
This question, seemingly simple, lies at the heart of how we think about AI at Menhir. Coming from an engineering background, we've always been uncomfortable with systems we don't fully understand. It turns out our customers feel exactly the same way.
Let me tell you a story about how this mindset led us to break open the AI black box, and in doing so, unlock an entirely new way of thinking about artificial intelligence in business.
The Limitation of ML Crystal Balls
A few years ago, we were working with a major European bank on their loan collections. Our AI system was accurately predicting which customers would default and which would pay. The predictions were strong – over 85% accurate. Yet something was missing.
The collections team wasn't fully acting on these predictions. When we dug deeper, we discovered why: they didn't just want to know what would happen; they needed to understand why it would happen and, more importantly, what they could do about it.
This was our lightbulb moment.
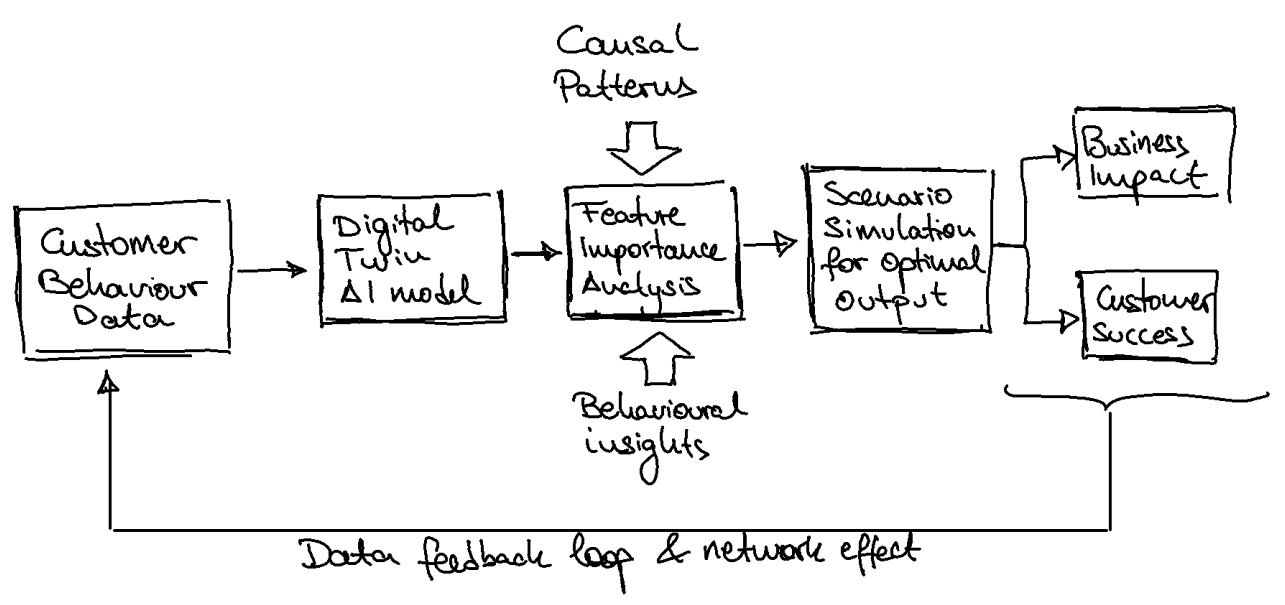
Breaking Open the Box
We realized that an AI prediction without explanation is like a diagnosis without symptoms – you might know what's coming, but you don't know how to prevent it. This led us to develop what we now call Customer Digital Twins – complete causal models of customer behaviour.
What is the opportunity?
Let's take a Churn Risk vs. Price Discount example:
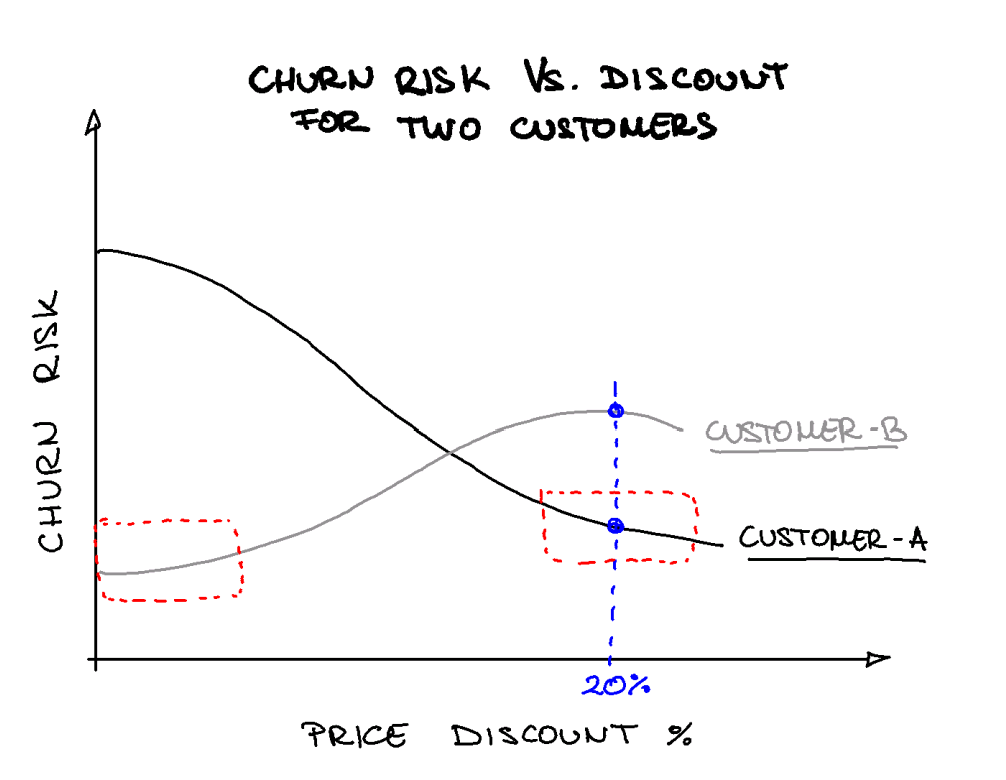
Let's say we launch a discount retention campaign. Customer A had a high risk of churn, and with the discount we keep the customer. On the other side, we have Customer B, who was not thinking about leaving the company, but as soon as he receives such an offer he starts thinking "am I overpaying?", thus, increasing the churn risk of a healthy customer.
Understanding this granularity and being able to act on these insights leads to one of the most powerful competitive advantages for companies leveraging huge volume of customers.
But we didn't stop there.
From Explanation to Action
The real breakthrough came when we started using these insights to simulate different interventions. Understanding the impact of the features we can act on, our system could now say:
- A 5% price adjustment would reduce churn risk by 40% for this given customer
- Proactive service contact could improve satisfaction by 25% for this other customer segment
- A product upgrade offer would be 60% likely to be accepted on this market
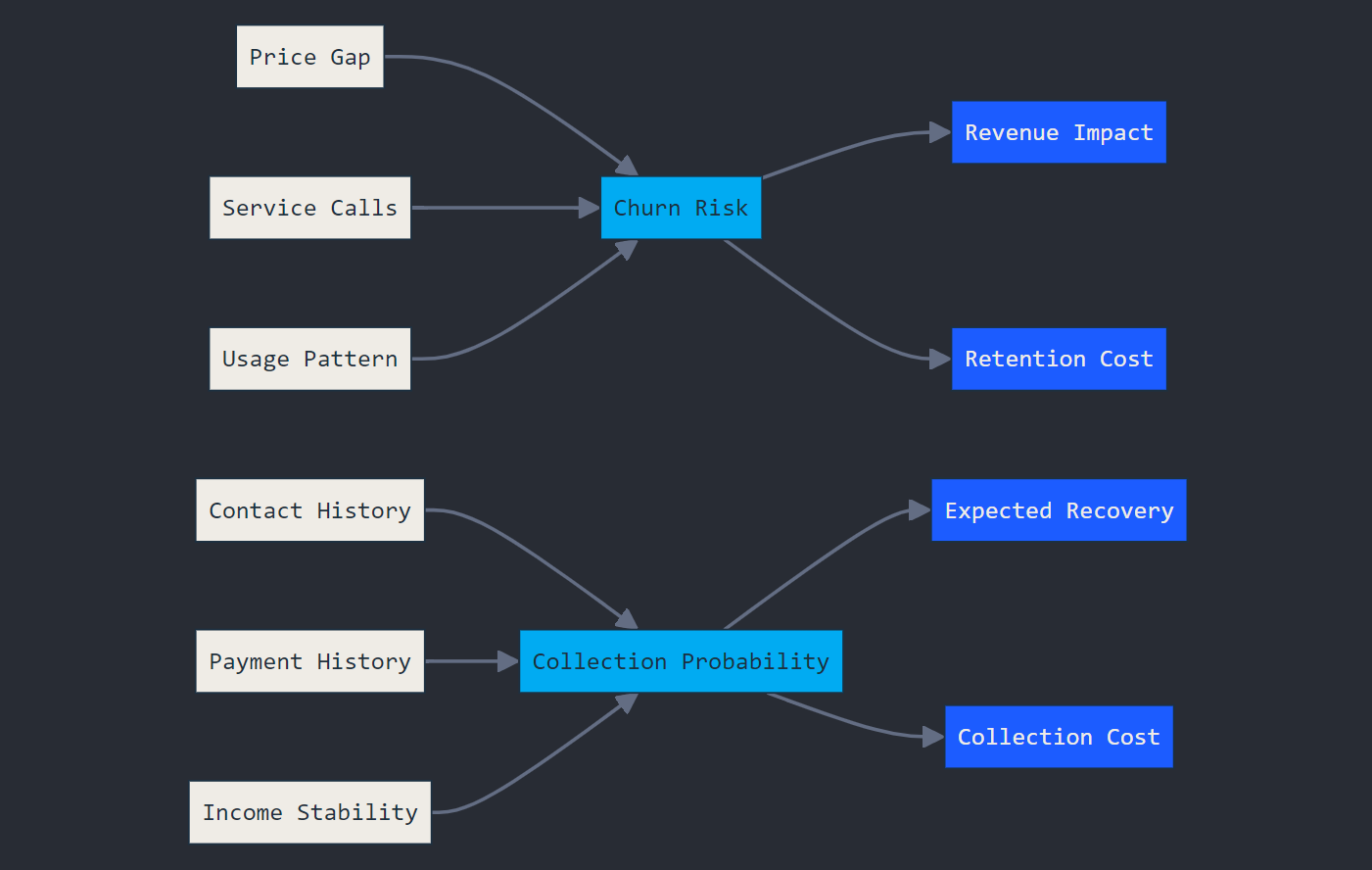
This wasn't just about understanding – it was about creating actionable pathways to better outcomes.
The Numbers Tell the Story
The impact was immediate and measurable. In loan collections:
- High-score loans showed a 22% collection rate
- Low-score loans remained at 10%
- The difference? Not just prediction, but understanding and targeted action
But the real magic happened when we applied this thinking across different industries:
In real estate, we discovered that property listings have an optimal description length – too short and they lack information, too long and they lose interest. But it wasn't just about length; it was about understanding why certain descriptions worked better than others.
In insurance, it is known that customer retention isn't just about price – it is about the interaction of multiple factors, each with its own threshold and impact level.
Beyond the Technical
This journey taught us something profound about AI in business: transparency isn't just about building trust – it's about enabling action. When people understand why an AI system makes certain predictions, they're more likely to:
- Trust the recommendations
- Take appropriate action
- Provide valuable feedback
- Improve the system over time
The Future of Explainable AI
What excites us most is where this is heading. We're moving beyond simple feature importance to understanding complex causal relationships. Imagine being able to:
- Create detailed simulations of customer behaviour
- Test interventions in a virtual environment
- Understand the ripple effects of each decision
- Optimize strategies before implementation
That's where Menhir AI is heading.
A New Paradigm
The black box era of AI is ending. Not because we don't need powerful AI systems, but because we need systems that think and explain, that predict and guide. Systems that don't just tell us what might happen, but help us understand how to shape what will happen.
This isn't just about making AI more transparent – it's about making it more useful, more actionable, and ultimately, more human.
Because in the end, the most powerful AI isn't the one that makes the best predictions; it's the one that helps us make better decisions.
Want to learn more about how explainable AI can transform your business? Let's talk